SMART detection and real-time learning in water distribution systems
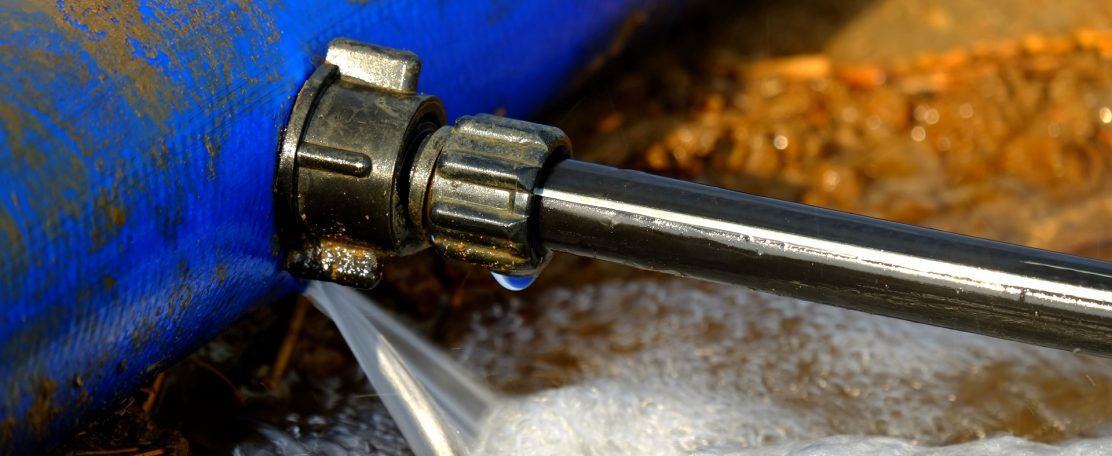
Ir. Doekle Yntema
Wetsus Theme Smart Water Grids
Dr.ir. Karel Keesman
Wageningen University / Wetsus, Mathematical and Statistical Methods
Prof.dr.ir. Jaap Molenaar
Wageningen University, Mathematical and Statistical Methods
Vitens, Evides, PWN, Brabant Water, Acquaint, Wavin, WUR, Wetsus
In areas with state-of-the-art drinking water production facilities and distribution systems, fresh drinkable water is well available and of excellent quality as a rule. However, there are situations where the water treatment and distribution process are disturbed due to technical failures, accidents or natural causes. Contamination of the drinking water due to these disturbances can seriously affect municipal life. In the worst case, hazardous components can reach the end-users. Detecting these disturbances quickly with proper mitigation strategies can minimize the impact.
Technology
For the multi-objective challenge of safe drinking water, robust maintenance and energy-efficient water supply, the integration of smart detection and alarming systems, including, amongst others, dense sensor networks, soft sensoring, real-time learning, hazard prediction, communication and mitigation strategies, in water distribution networks are indispensable. Most issues in drinking water distribution networks are covered well with standard detection techniques. Still, it is possible to obtain much more information from existing sensor data when combined and processed in real time. This way, the resulting information per sensor is much higher since additional information is obtained from the relation between sensors.
Challenge
The specific problem tackled in this project is how to process the noisy and perturbed real-time data streams from the water distribution sensor networks for pattern recognition, optimization of the sensor network, correction of missing data (back-casting) and increase of intelligence in the network, leading to better (automated) management.
Solutions
To tackle the problem of real-time learning and early detection for safe, robust and energy-efficient water supply from noisy and perturbed data, the following steps will be made:
- Collection of real-time data sets from Vitens’ distribution network (demo site NE Friesland)”;
- Inventory of the data sets –missing data, need for additional data;
- Cross-correlation and (cross)spectral analyses of the data sets to discover “unexpected” relationships, using modern techniques such as FFTW (fast Fourier transform in the west) and SVM (support vector machines);
- Analysis and synthesis of novel recursive correlation, regression and spectral methods for learning in a real-time environment, thus allowing immediate updates of states and flows;
- Integrated data–model analysis for understanding the dynamics of the distribution network and detecting deficiencies of the existing sensor network, possibly leading to a redesign of the sensor network;
- Testing of prototype software under real-world conditions, thus with real-time data as provided by Vitens (demo site NE Friesland) and preferably other distribution networks, for the prediction of abnormalities as a first but relevant step towards more efficient maintenance;
- Evaluation of the results from the step for further understanding of the dynamic behaviour of water distribution networks.
Wetsus
2017WET003